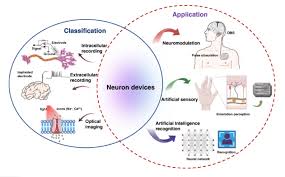
Nanomaterials for Next-Generation Neuromorphic Applications
Neuromorphic engineering, an interdisciplinary field that combines neuroscience and electrical engineering, aims to create systems that mimic the way biological brains process information. This approach promises to revolutionize computing, making it faster, more efficient, and capable of performing complex tasks such as pattern recognition, learning, and sensory processing. A key player in advancing neuromorphic applications is the use of nanomaterials, which offer unique properties that enhance the performance of neuromorphic devices. These materials are essential for developing brain-like computing systems that can perform real-time, energy-efficient cognitive tasks.
1. What are Neuromorphic Systems?
Neuromorphic systems are designed to emulate the neural structures and functions of the human brain. These systems often utilize artificial neurons and synapses that process information in a way that is similar to how biological neurons interact with each other. Traditional computing systems, in contrast, rely on binary logic gates and processors that require high amounts of energy for processing and storage.
Neuromorphic computing systems are more flexible, adaptive, and energy-efficient. They aim to enable machines to learn from experience, recognize patterns, and make decisions without relying on a centralized processor or excessive memory storage. For these systems to function optimally, their hardware needs to exhibit properties such as high connectivity, synaptic plasticity, and low energy consumption.
2. Role of Nanomaterials in Neuromorphic Applications
Nanomaterials, with their unique physical, chemical, and electrical properties, are well-suited to address the challenges faced by traditional computing systems. Their nanoscale size allows for high density, precise control over material properties, and the ability to manipulate individual atomic or molecular configurations, which is essential for mimicking brain functions.
The key roles that nanomaterials play in neuromorphic applications include:
A. Synaptic Mimicry
One of the most critical aspects of neuromorphic computing is the ability to replicate the behavior of biological synapses, which are the junctions between neurons that transmit signals. Synaptic plasticity, the ability of synapses to strengthen or weaken over time, is a fundamental mechanism of learning and memory.
Nanomaterials, such as transition metal oxides, carbon-based nanomaterials, and 2D materials, have been explored for synaptic mimicry due to their ability to undergo reversible changes in conductivity, similar to how biological synapses adapt to stimuli. This property is vital for creating artificial synapses that can store and process information in a way that emulates biological learning processes.
For example, memristors, which are often made from nanomaterials, exhibit the ability to retain resistance based on the history of voltage applied, mirroring synaptic strength changes. This makes them promising candidates for building neuromorphic devices capable of learning and adapting to new information in real time.
B. Energy Efficiency
One of the biggest advantages of nanomaterials in neuromorphic systems is their potential for ultra-low energy consumption. Nanotransistors and other nanoscale devices operate at lower voltages and currents, reducing the power required for computations. This is essential for neuromorphic applications, where energy efficiency is paramount for long-term performance, particularly for portable or wearable devices.
Nanomaterials can also be engineered to take advantage of quantum effects, such as tunneling, which allow for even greater energy efficiency in neuromorphic systems. By leveraging the unique properties of nanomaterials, such as their high surface-to-volume ratio and tunable electrical characteristics, neuromorphic circuits can be made smaller and more energy-efficient compared to traditional electronic systems.
C. High-Density Integration
Another crucial characteristic of nanomaterials is their ability to enable high-density integration. In the human brain, billions of neurons and trillions of synaptic connections operate simultaneously, enabling complex information processing. For neuromorphic systems to replicate this scale, they must be able to integrate millions of artificial neurons and synapses in a small, efficient package.
Nanomaterials, such as nanowires, nanotubes, and graphene, can be used to create compact, highly conductive pathways for information transmission. These materials allow for a denser arrangement of artificial neurons and synapses, making them ideal for scalable, brain-like computing systems.
D. Flexibility and Adaptability
Nanomaterials exhibit high levels of structural flexibility and adaptability. This makes them suitable for designing neuromorphic systems that can mimic the plasticity of the brain. The ability of nanomaterials to change their structure in response to external stimuli is crucial for neuromorphic devices that need to learn from experience and adapt to new inputs.
For example, nanomaterial-based transistors can change their conductance based on the input received, enabling the system to “learn” patterns or behaviors. This feature is similar to how biological neurons form and adjust synapses in response to stimuli, allowing artificial systems to improve their performance over time.
3. Types of Nanomaterials Used in Neuromorphic Applications
Several types of nanomaterials are being investigated for their potential use in neuromorphic applications. These include:
A. Carbon Nanotubes (CNTs)
Carbon nanotubes are cylindrical nanostructures made of rolled-up graphene sheets. Their high electrical conductivity, mechanical strength, and scalability make them ideal for creating nanoscale transistors and artificial synapses in neuromorphic systems. CNTs are also highly flexible and can be used in stretchable electronics, which is beneficial for applications in wearable or flexible neuromorphic devices.
B. Memristors
Memristors are passive two-terminal devices that can store information as resistance, similar to the way biological synapses store information as synaptic strength. These devices can be made from a variety of nanomaterials, such as metal oxides, graphene oxide, and carbon-based materials. Memristors are particularly useful in synaptic emulation because they exhibit non-volatile memory, meaning they can retain their information even when the power is turned off.
C. 2D Materials
2D materials like graphene, transition metal dichalcogenides (TMDs), and black phosphorus are being explored for neuromorphic applications due to their outstanding electrical properties, high surface area, and scalability. These materials can be used to construct artificial neurons, synapses, and high-performance transistors in neuromorphic circuits.
D. Nanowires and Nanorods
Nanowires and nanorods made from materials like silicon, gold, or silver are being used to create highly conductive pathways in neuromorphic circuits. These materials allow for the construction of dense, high-speed interconnects that are essential for replicating the connectivity of biological neural networks.
4. Challenges and Future Outlook
While nanomaterials hold tremendous potential for neuromorphic applications, several challenges remain. These include:
- Scalability: Producing nanomaterials at a large scale for practical neuromorphic systems is still a major challenge.
- Stability: The long-term stability and reliability of nanomaterials under real-world conditions need to be addressed before they can be used in commercial applications.
- Integration: Integrating nanomaterials into existing electronic systems and ensuring they work seamlessly with traditional semiconductor devices remains an ongoing challenge.
However, ongoing research and development in the field of nanomaterials, coupled with advancements in nanofabrication techniques, are likely to lead to the commercialization of neuromorphic systems that are not only powerful but also energy-efficient and scalable.
5. Conclusion
Nanomaterials are poised to play a pivotal role in advancing neuromorphic computing, offering new opportunities for brain-like processing, adaptive learning, and energy-efficient systems. By leveraging the unique properties of materials such as carbon nanotubes, memristors, and 2D materials, researchers are creating the next generation of neuromorphic devices that can mimic the learning and processing capabilities of the human brain. While challenges remain, the future of neuromorphic applications powered by nanomaterials holds immense potential across a wide range of industries, from artificial intelligence to healthcare.